Our library homepage‘s main search area currently looks like this:
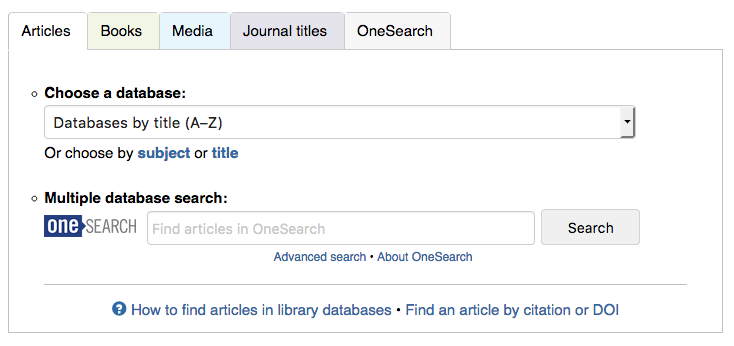
The tab box has served us well for years, but it’s about time we upgraded to something simpler and easier to use. We plan to give our entire website a nice update over the summer, mostly subtle aesthetic changes, but with a big search box upgrade. We’re currently in a discussion about whether to keep the general idea of tabs (expecting users, mostly students, to know ahead of time whether they’re looking for articles or books) or to present one big OneSearch box (à la Google).
TL;DR: We plan to present the big-box search that students say they love, but include Article/Book/Media scopes as a didactic hint and visual cue. This post looks at how other libraries present scope options, if any.
Our website analytics tell us that students mostly use the scoped options. In the tab box, the 4 highest-use options, in order: database dropdown menu, OneSearch box scoped to articles, OneSearch box scoped to books, and OneSearch box non-scoped. This is strongly influenced by the options that we present to the user.
Do we keep these scopes in our redesign? Students compose most of our users. On the one hand, they’ll probably say they’d prefer a big search box, like Google and Amazon. On the other hand, when they approach the reference desk, they’ll usually say, “I’m looking for a book called…” or “I need to find articles on…” So what do we do?
Step 1: Let’s see what other libraries are doing.
Search UIs within CUNY
Just about every CUNY defaults to the same discovery layer, OneSearch (aka Primo, the web-scale discovery system from Ex Libris), along with offering a handful of databases and other resources that are campus-specific. OneSearch has the same scope options for every CUNY campus.
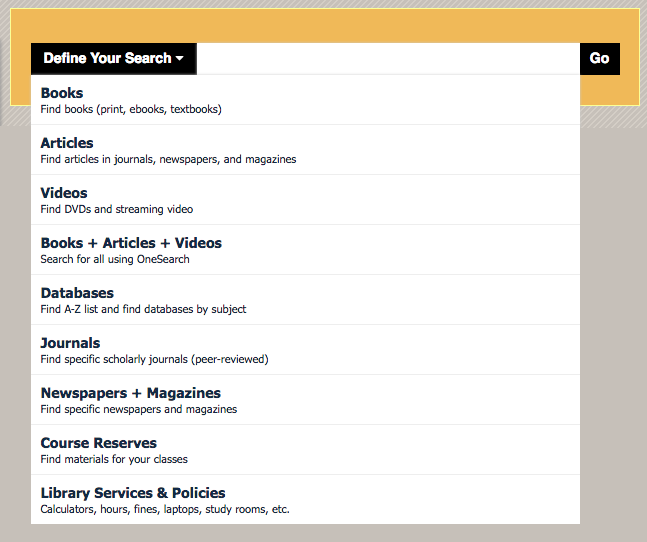
The Newman Library at Baruch College requires the user to select a scope first before they can type in the search box. Some of these options are links (like Databases) rather than ways to scope the search.
—

Hunter College Libraries default to OneSearch, but offers faded tabs for optional scoping. Media (videos, mostly) isn’t a scope they offer.
—
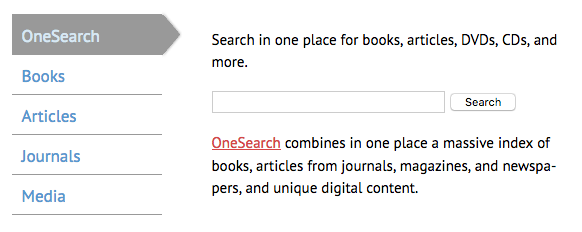
The College of Staten Island Library does the same as Hunter, but with vertical tabs.
—

The Schwerin Library at City Tech has a dropdown of scopes, but defaults to a non-scoped OneSearch. Interestingly, there are three options for books: print, ebooks, and both. (Here at John Jay, most students seem to prefer print books, so they’d probably appreciate this option.) Databases are linked outside of the tab box.
—
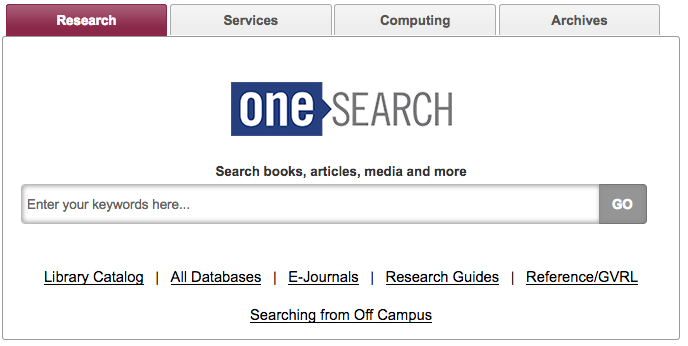
Brooklyn College Library offers just one search box, for a non-scoped OneSearch search, but provides text links to CUNY+ and databases below.
—
In fact, all CUNY libraries’ approaches to OneSearch were tabulated and analyzed by Nora Almeida, Helen Georgas, and Alexandra Hamlett (all CUNY librarians) for their “The Cosmography of Discovery: Integration, Student Perceptions, and Information Design” presentation at the ER&L 2017 Conference. (If you/your institution has an ER&L Conference login, you can watch their presentation slides + audio — highly recommended!)
In looking at the above sampling, I also notice that John Jay is an outlier for offering a shortcut list of “popular” databases, rather than directing users to the full list of databases, which can number over 100. This campus is pretty tied to that list of popular databases, and I think it’s a good idea to keep that shortcut. (Even if it might diminish the use of the “non-popular” databases.)
Other interesting search UIs
Outside of CUNY, here are a few of my fave academic library websites:
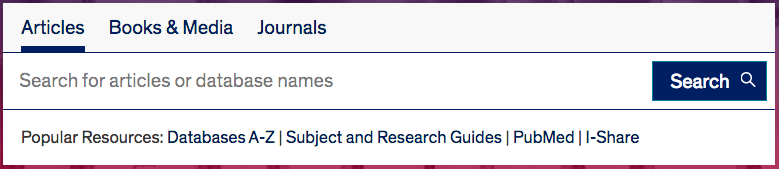
The University of Illinois—Chicago Library search box is currently the above, but apparently they’ll soon be moving to a “Search Everything” bento-box search UI. (I wonder if it really is “everything”?)
The look of the tab box is very clean and technical, mirroring the look of the UIC site as a whole.
A 2017 Code4Lib article, “Participatory Design Methods for Collaboration and Communication,” by Tara M. Wood and Cate Kompare, goes behind the scenes for the UIC website redesign. It’s a fantastic article.
—
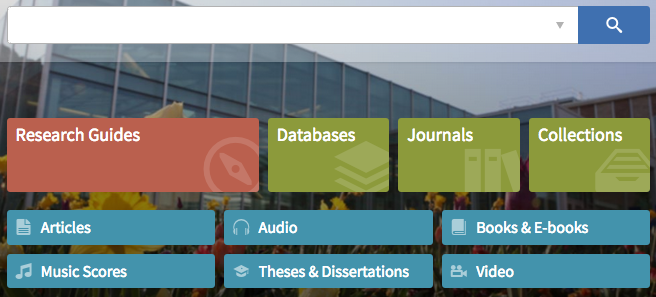
The BYU Library has an unlabeled search box for their discovery system (with an advanced search option subtly indicated), and includes lovely buttons below advertising specific resources and majorly highlighting their libguides.
—

The library at my alma mater, Brown, just has one big search box in the middle of the screen. It’s actually unusual how prominently that box is placed. They’ve put other options below the fold:

—
Trying a new tab box
So it seems that most other libraries default to an “everything” search, but offer scope options. That seems like it might be a good solution for us, too, but John Jay students are a unique group. What will work for them?
Here at John Jay, I’m now playing with two mocked-up widget boxes. Here’s the first, strongly inspired by UIC:

Defaults to non-scoped OneSearch, as most other CUNYs seem to do, but gives the option for Articles (and peer-reviewed articles), Books, and Media. This, to me, seems like a natural next step: Presenting the big-box search that students say they love, but prominently displaying scope options as a didactic hint and visual cue. This gives the user a hint as to what OneSearch includes. Will students actually use these scopes? We’ll have to test to find out.
One big issue with the “peer-reviewed” checkbox is that OneSearch can only narrow results down to articles published in peer-reviewed journals, but not necessarily peer-reviewed articles, so reviews and columns will show up in the results. We discussed this in our Web Committee, and this is apparently a problem with pretty much all discovery systems, so… Oh, well, we’ll just live with that? I know many students will appreciate this kind of filter, even if it’s problematic. We can use explanatory hover text.
Another issue: “Articles” is distinct from “newspaper articles” as a scope in OneSearch. To sort-of-solve this, I put more explanatory hover text over Articles. (Technically, you can cobble together multiple after-search facets, which Baruch does, but then when the user changes their keywords and searches again, after-search facets disappear, which we didn’t like at John Jay. So we stick to pre-search scopes, which can’t be combined, but do stick around after a keyword revision.)
This tab box includes Databases as a top-level tab:

Database-specific searching is still how we teach some classes and how many students and faculty alike prefer to search. The tab includes the Popular Dropdown menu, which also directs users to browsing databases by title/subject (duplicating the links below the dropdown menu).
—
Another tab box I messed around with, more similar to our current one, and inspired by Baruch:

The dropdown menu opens to: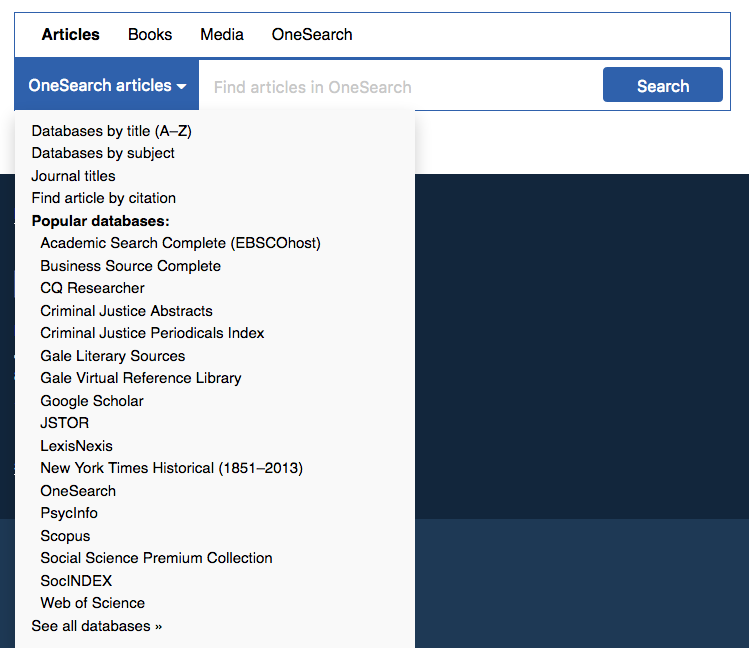
Here, OneSearch is the default search for every tab, and only Articles has any other option. Inspired by Baruch, I faked parts of the dropdown menu — that is, clicking JSTOR won’t present a JSTOR-only search box, it will just open JSTOR as a link.
—
In a recent Web Committee meeting with other John Jay librarians, we agreed to pursue the first tab box, but perhaps with refinements. Next week, we’ll do some guerrilla usability testing outside the cafeteria so that we can see whether students find the new tab more or less usable. Stay tuned!